Keynote speakers
Implicit Neural Representations Meet Semantic Communications
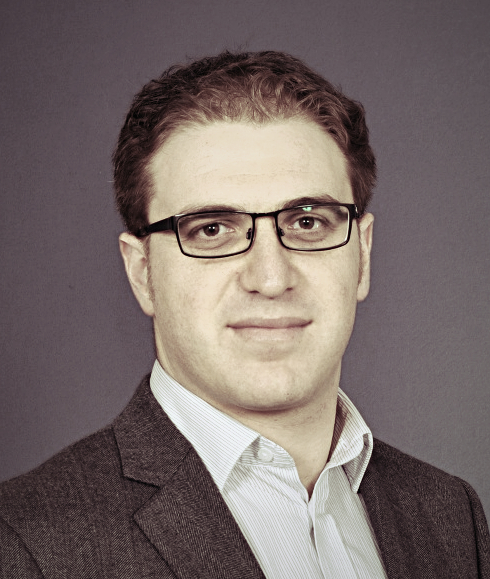
Short Biography
"Deniz Gündüz earned his Ph.D. from the NYU Tandon School of Engineering in 2007, where he has since made significant contributions to his field. In 2012, he joined the Electrical and Electronic Engineering Department at Imperial College London, UK, where he is currently a Professor of Information Processing, and serves as the deputy head of the Intelligent Systems and Networks Group. In the past, he held positions at the University of Modena and Reggio Emilia (part-time faculty member, 2019-22), University of Padova (visiting professor, 2018, 2020), Centre Tecnologic de Telecomunicacions de Catalunya (CTTC) (research associate, 2009-12), Princeton University (postdoctoral researcher, 2007-09, visiting researcher, 2009-11) and Stanford University (research assistant professor, 2007-09). His research interests lie in the areas of information theory, machine learning, wireless communications and privacy. Dr. Gündüz is a Fellow of the IEEE. He is an elected member of the IEEE Signal Processing Society Signal Processing for Communications and Networking (SPCOM) and Machine Learning for Signal Processing (MLSP) Technical Committees. He chairs the UK and Ireland Chapter of the IEEE Information Theory Society, and serves as an area editor for the IEEE Transactions on Information Theory. In the past, he served in editorial roles for the IEEE Transactions on Communications, IEEE Transactions on Wireless Communications, IEEE Journal on Selected Areas in Communications and IEEE Journal on Selected Areas in Information Theory. He is the recipient of the IEEE Communications Society - Communication Theory Technical Committee (CTTC) Early Achievement Award in 2017, Starting (2016), Consolidator (2022) and Proof-of-Concept (2023) Grants of the European Research Council (ERC), and has co-authored several award-winning papers, most recently the IEEE Communications Society - Young Author Best Paper Award (2022), and the IEEE International Conference on Communications Best Paper Award (2023). He received the Imperial College London - President's Award for Excellence in Research Supervision in 2023.
Abstract
As deep neural networks (DNNs) continue to revolutionize computing, a critical challenge emerges: how can we efficiently deliver these increasingly large models over bandwidth-constrained mobile networks? This challenge is particularly pressing as state-of-the-art DNNs become more specialized for specific times, locations, and tasks, requiring on-demand delivery to users' devices. Similarly, distributed learning scenarios require frequent exchange of model parameters across wireless networks. I will highlight that these problems are examples of `semantic communications’, where the goal is not to recover network parameters reliably, but to make sure they serve for the desired task at the receiver.
In this talk, I will first present our recent breakthroughs in efficient DNN parameter compression and transmission over wireless channels, demonstrating how these techniques can reduce bandwidth requirements while preserving model performance. Building on these foundations, I will then explore how our techniques extend naturally to implicit neural representations (INRs), which treat signals as continuous functions represented by neural networks. This innovative approach bridges traditional signal processing with modern deep learning, yielding remarkable results for practical applications. Specifically, I'll demonstrate how our INR-based methods achieve state-of-the-art performance in MIMO channel state feedback and image compression tasks, reducing transmission overhead while maintaining signal fidelity.
Zero-touch Networks: Past, Present, and Future
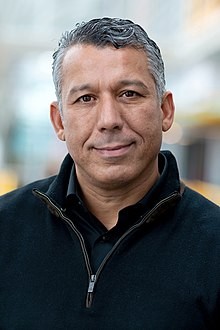
Raouf Boutaba
David R. Cheriton School of Computer Science
University of Waterloo
Canada
Short Biography
Raouf Boutaba is a University Professor and the Director of the David R. Cheriton School of Computer Science at the University of Waterloo. Before that he served as the Associate Dean Research (2016-2019) and the Associate Dean Innovation and Partnership (2019-2020) in the Faculty of Mathematics. Between 2017 and 2022, he held an INRIA International Chair in France and currently holds a University Chair and the Rogers Chair in Network Automation at Waterloo. His research interests are in the areas of network and service management. He has published extensively in this are and received over 20 journal and conference and journal best paper awards, including the IEEE CNOM test of time award. He is the founding Editor-in-Chief of the IEEE Transactions on Network and Service Management (2007-2010) and served as the Editor-in- Chief of the IEEE Journal on Selected Areas in Communications (2018-2021). He received several awards, including the Silver Core from IFIP, the Hal Sobol, the Salah Aidarous, the Joe LociCero, the Dan Stokesbury, and the Donald W. McLellan awards from the IEEE Communications Society, and the McNaugthon Gold Medal from IEEE Canada. He is fellow of the IEEE, the Engineering Institute of Canada, the Canadian Academy of Engineering, and the Royal Society of Canada.
Abstract
Automation has been the holy grail of network management research fordecades. It aims at achieving autonomous networks, i.e., networks thatare capable of independently monitoring their status, analyzingproblems, making decisions, and executing corrective actions. Despiteseveral attempts to achieve autonomous networks in the past, theirpractical deployments have largely remained unrealized. Several factorscontribute to this, including the existence of many stakeholders withconflicting objectives, reliance on proprietary solutions, the inabilityto process network monitoring data at scale, and the lack of globalvisibility restricting network-wide optimizations. The stars are nowaligned to realize the vision of network automation, driven by: (i)advances in network softwarization, (ii) recent breakthroughs in machinelearning, and (iii) the availability of large-scale data processingplatforms. However, several challenges remain in creating synergy acrossthese different technology domains. With renewed interest in autonomousnetworks, particularly the push towards zero-touch paradigms as afoundation for Beyond 5G (B5G) and 6G network automation, this keynotewill explore the past, present, and future of autonomous networks,highlight key challenges impeding their realization, and discusspotential solutions.